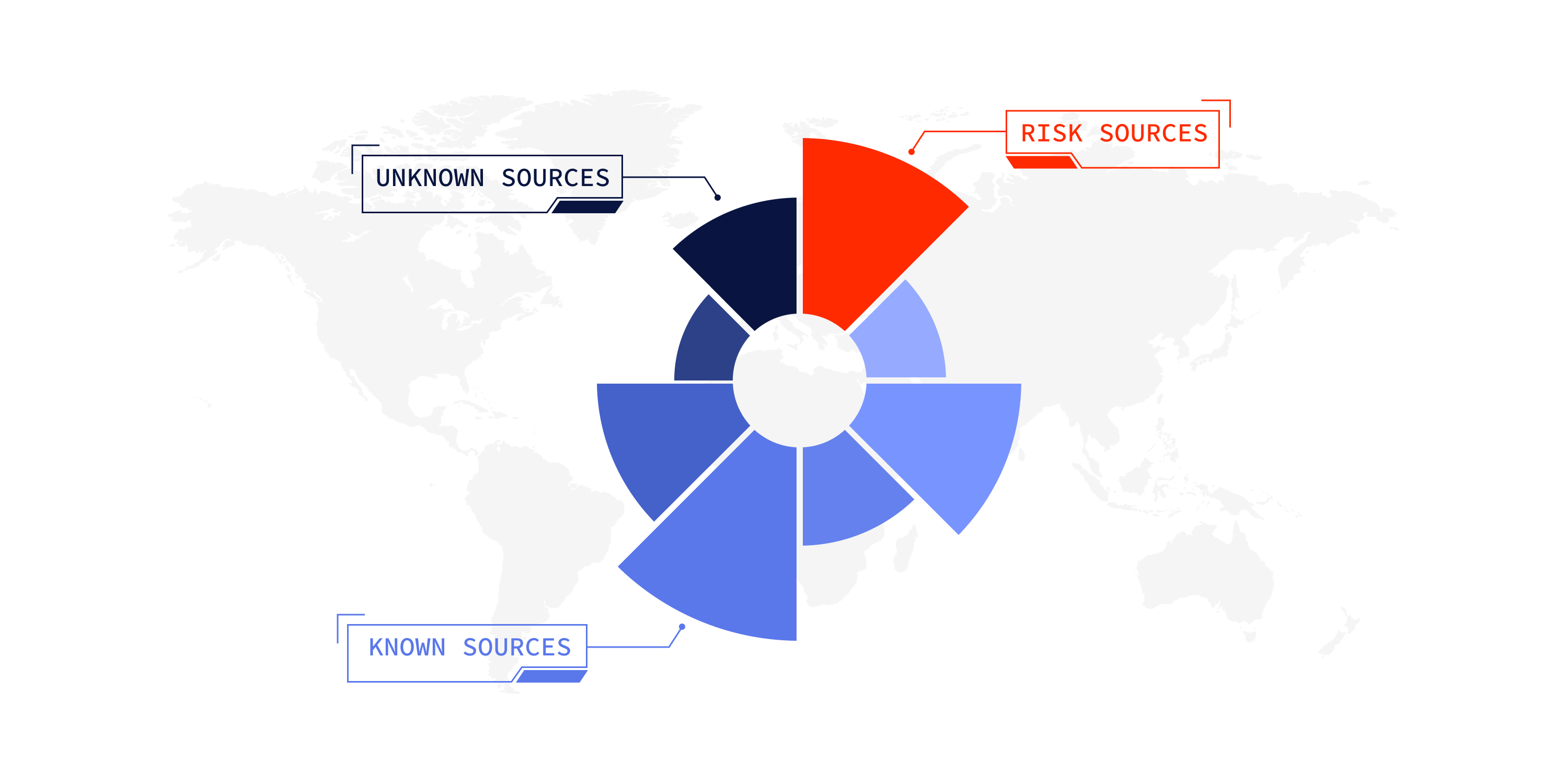
What is the concept of a risk-based approach?
The risk-based approach encompasses the steps taken by financial institutions or cryptocurrency companies to identify the most critical compliance risks for their organization. A risk-based approach is a fundamental principle outlined in the Financial Action Task Force (FATF) guidelines regarding virtual assets. This approach aims to ensure that businesses and financial institutions identify, assess, and mitigate the risks associated with money laundering and terrorist financing effectively. By adopting a risk-based approach, the processes for assessment, monitoring, managing, and mitigating risks associated with money laundering and terrorist financing, organizations can enhance their ability to combat illicit activities within the virtual asset space. Let’s dive into and understand why these processes are so important.
Risk Assessment Process
In accordance with the Financial Action Task Force (FATF) guidelines, it is imperative to conduct a comprehensive evaluation of money laundering and terrorist financing (ML/TF) risks associated with cryptocurrency transactions. This assessment should consider various factors, including the types and origins of virtual assets involved, transaction volumes, customer profiles, and geographic locations. Furthermore, it is essential to identify ML/TF indicators specific to the crypto industry, such as large or excessively frequent transactions, engagement with high-risk jurisdictions like Iran, Democratic People’s Republic of Korea, and Myanmar (listed on the FATF “blacklist”), utilization of anonymity-enhancing technologies (AETs) like mixing services or privacy coins, as well as transactions linked to known criminal entities or sanctioned individuals. The assessment should also encompass the evaluation of risks arising from interactions with third parties.
Regulatory compliance program
To adhere to FATF guidelines, it is vital to develop a comprehensive compliance program that delineates policies, procedures, and control measures aimed at mitigating ML/TF risks. This program should encompass internal controls, employee training, independent audits, and the appointment of a designated compliance officer, such as the MLRO, responsible for overseeing the effectiveness of the program.
Customer Due Diligence (CDD)
Establishing robust CDD procedures is a fundamental requirement outlined by FATF. This entails verifying customer identities, evaluating their risk profiles, and gaining an understanding of the nature of their crypto transactions. To achieve regulatory compliance measures such as Know Your Customer (KYC) checks, enhanced due diligence for high-risk customers, and ongoing monitoring of customer activities must be implemented. During the onboarding process, it is crucial to conduct comprehensive screenings of customers against Politically Exposed Persons (PEP) and sanctions lists. These lists consolidate regulatory and enhanced due diligence data from major sanctioning bodies worldwide, such as the Office of Foreign Assets Control (OFAC), UN sanctions, EU sanctions, His Majesty’s Treasury, Interpol, and numerous other regulatory and law enforcement organizations. This enables the identification of individuals with potential political influence or involvement in illicit activities.
Transaction Monitoring
A robust system for monitoring and analyzing crypto transactions should be established to detect suspicious activities. Transaction monitoring systems must be capable of identifying potential ML/TF red flags, such as structured transactions, round-trip transactions, unusual transaction volumes, and other suspicious behaviour. Employing behavioural analysis techniques to identify abnormal transaction patterns and deviations from expected behaviour is recommended.
Ongoing Monitoring and Review
Customer profiles are subject to change over time, necessitating continuous monitoring efforts. Regularly reviewing transactions for signs of criminal activity is crucial. Additionally, anti-fraud alerts should be processed, such as detecting extended logins from restricted countries or multiple users sharing a single wallet (as a pattern of third-party transactions). Furthermore, inconsistencies in a client’s profile, such as a significant deposit despite regular income, should be promptly identified and investigated.
Reporting and Record-Keeping
Compliance with local regulations necessitates the establishment of mechanisms for reporting suspicious transactions to the relevant authorities. Seated compliance officers, such as the Money Laundering Reporting Officer (MLRO), must file Suspicious Activity Reports (SARs) with the appropriate regulator and law enforcement agencies whenever suspicious activity is detected. These reports should be timely, accurate, and contain all the necessary information to facilitate investigations and law enforcement actions. All records and documentation pertaining to customer due diligence, transactions, and risk assessments should be diligently maintained, ensuring ease of access for regulatory inspections.
Global Ledger blockchain analytics tools make it possible to easily apply the FATF standards in the field of risk assessment and transaction monitoring of the risk of base approach to the everyday routine of compliance teams. The toolset serves government agencies, banks, fintech companies, and crypto startups, facilitating their compliance efforts in the realm of anti-money laundering.
But how does the GL toolset do it?
The GL toolset helps to build and implement a system for monitoring, risk assessment and analyzing crypto transactions to detect suspicious activity. The basic principle of the GL system can be described as follows – the more risk sources – the higher the risk score.
The main GL calculation algorithm is based on searching for sources of funds from the last incoming transactions by the requested address.
The algorithm analyzes only those paths that have contributed to the requested address. The share (%) of each source is calculated from the total amount of incoming funds of considered transactions. Found sources are counted, grouped, and displayed in the doughnut charts and tables. Each found source has a score.
What is the “score” in the GL toolset?
The score is a value from 0 to 100, which is needed to show the degree of risk.
- 0 – lowest risk (green colour)
- 50 – medium risk (yellow colour)
- 100 – highest risk (red colour).
Score value belongs to types and tags that are assigned to the found entity.
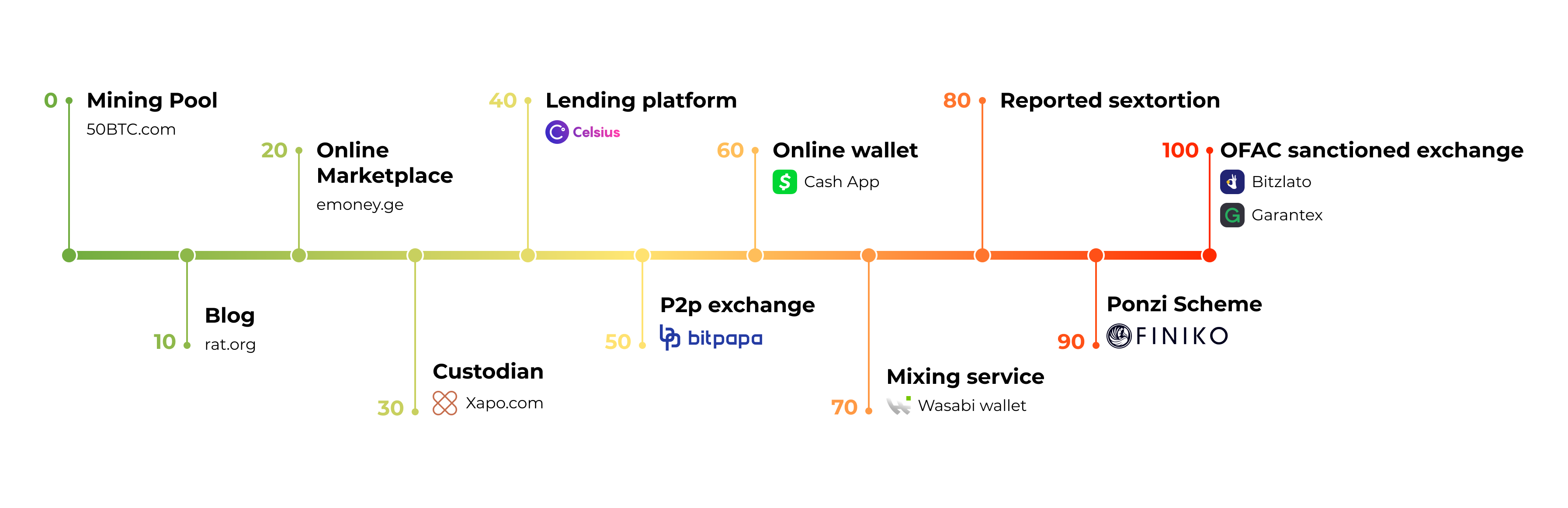
Score of source
The algorithm determines the source score by selecting the tag or types with the highest score assigned to the source.
Let this be illustrated by real-world examples:
CASE 1
The hacker’s wallet (score of 100) is in the Coinbase cluster (score of 5).
The funds from the hacker’s wallet and from the Coinbase cluster are counted separately because the address has a higher score than the cluster.
CASE 2
Miner’s wallet (with a score of 0) is in the Darkweb cluster (score of 100).
The funds from the miner’s wallet and from the Darkweb cluster are counted together, because the cluster has a higher score than the address.
What is a Risk Score?
The risk score is a value that calculates by the GL algorithm and is based on:
- analysis of the sources of funds received to the wallet address that is being checked, the types of these funds, their tags, as well as the AML risks of all counterparties
- contributed amount to the requested address
- depth* of the found source
*If the algorithm does not find any of the stopping conditions on the calculated transaction, it moves on to the previous transaction(s) and checks its inputs for the stopping conditions.
To avoid an endless search, the algorithm is tracking the number of transitions made to the previous transaction(s) in the source search – DEPTH.
In most cases, this is the number of “manual transitions” from the requested transaction to the found source.
Each transition to the previous transaction(s) is valued as 1 unit of depth.
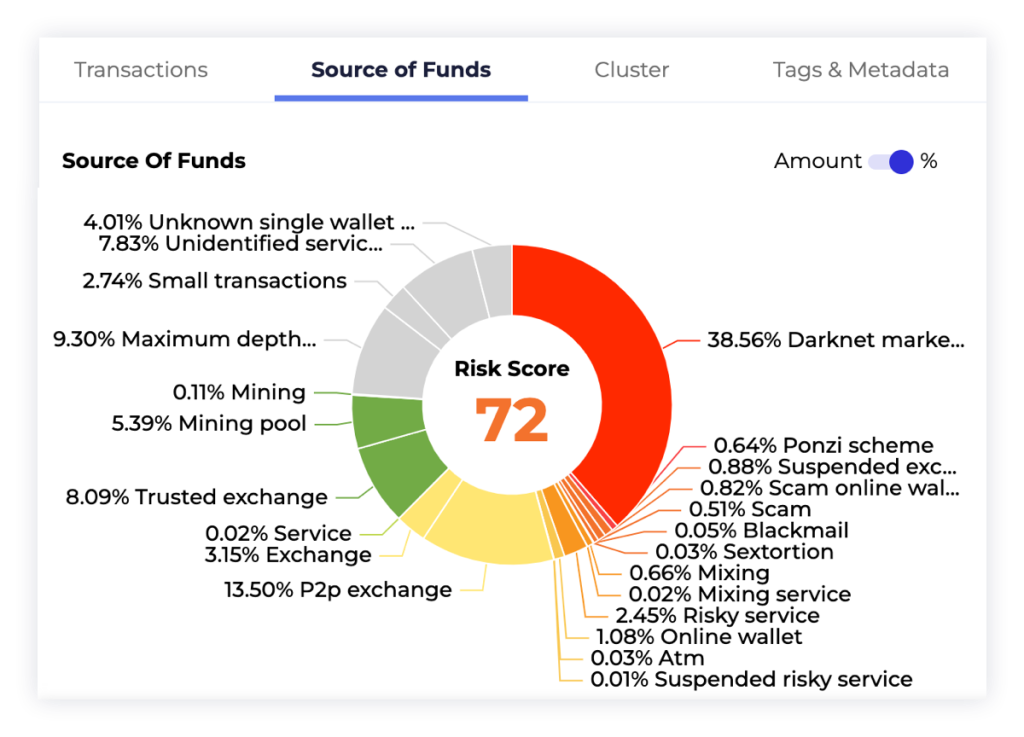
The risk scoring algorithm assigns greater significance to sources that pose higher risks, recognizing their increased importance to the compliance officer.
Users have the ability to choose multiple risk formulas and customize their risk tolerance levels in relation to different companies. Moreover, the GL toolset incorporates an ultra-high risk flag feature, which assigns a remarkably high risk score to sensitive sources such as sanctioned entities, even with minimal contributions. This caters to users who place great importance on avoiding any involvement with illicit funds originating from specific addresses.
By adopting a risk-based approach, organizations can focus their resources on the areas that pose the highest risk, allowing for a more targeted and efficient approach to combating illicit activities within the virtual asset space. This approach contributes to the overall goal of maintaining the integrity and security of the global financial system in the context of virtual assets. Leveraging the capabilities of the GL toolset, government agencies, banks, fintech companies, and crypto startups can enhance their compliance efforts in money laundering and terrorist financing activities in the crypto space.